https://scikit-learn.org/stable/datasets/toy_dataset.html
7.1. Toy datasets
scikit-learn comes with a few small standard datasets that do not require to download any file from some external website. They can be loaded using the following functions: These datasets are usefu...
scikit-learn.org
sklearn에서 기본적으로 제공되는 6가지의 데이터셋입니다.
1. 붓꽃 (iris) 데이터 (load_iris, 분류 문제)
- 붓꽃 분류를 다루는 데이터입니다.
- target 3개: 0: Setosa, 1: Versicolor, 2: Virginica (각 붓꽃의 종류)
- 변수 4개:
- 'sepal length (cm)', 'sepal width (cm)', 'petal length (cm)', 'petal width (cm)'
- (sepal (꽃받침) 길이, 넓이 (cm))
- (petal (꽃잎) 길이, 넓이 (cm))
import pandas as pd
from sklearn.datasets import load_iris
iris = load_iris()
df = pd.DataFrame(iris.data, columns=iris.feature_names)
df['target'] = iris.target
df.head()
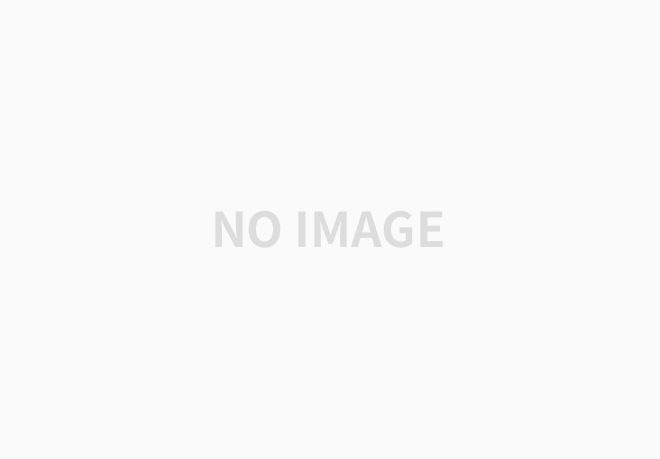
2. 당뇨병 (diabetes) 데이터 (load_diabetes, 회귀 문제)
- 당뇨병 수치를 다루는 회귀 데이터입니다.
- target: 25~346
- 변수 10개 (각 변수 값을 -0.2 (최소)와 0.2 (최대)사이 값으로 변환):
- 'age', 'sex', 'bmi', 'bp', 's1', 's2', 's3', 's4', 's5', 's6'
- (연령, 성별, BMI 지수, 혈압, s1~s6)
- s1~s6: 총 혈청 콜레스테롤, 저밀도 지단백질, 고밀도 지단백질, 총 콜레스테롤 / 고밀도 지단백질, 혈중 트리글리세라이드 수준의 로그, 혈당 수준
import pandas as pd
from sklearn.datasets import load_diabetes
diab = load_diabetes()
df = pd.DataFrame(diab.data, columns=diab.feature_names)
df['target'] = diab.target
print(df.shape)
df.head()
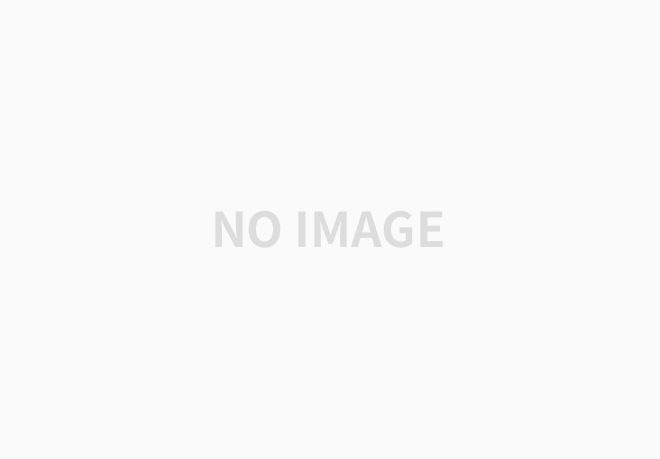
3. 손글씨 숫자 데이터 (load_digits, 분류 문제)
- 0부터 9까지의 손글씨 숫자를 분류하는 데이터입니다..
- target 10개: 0~9
- 변수 64개: 총 64개의 픽셀 (0 0, 0 1, ... 7 7)
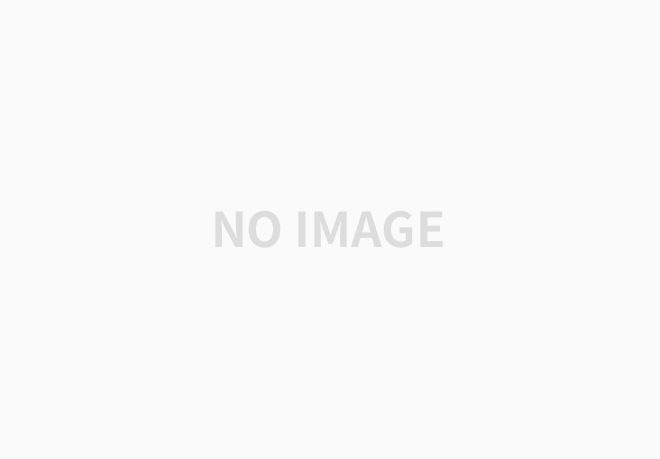
import pandas as pd
from sklearn.datasets import load_digits
digits = load_digits()
df = pd.DataFrame(digits.data, columns=digits.feature_names)
df['target'] = digits.target
print(df.shape)
df.head()
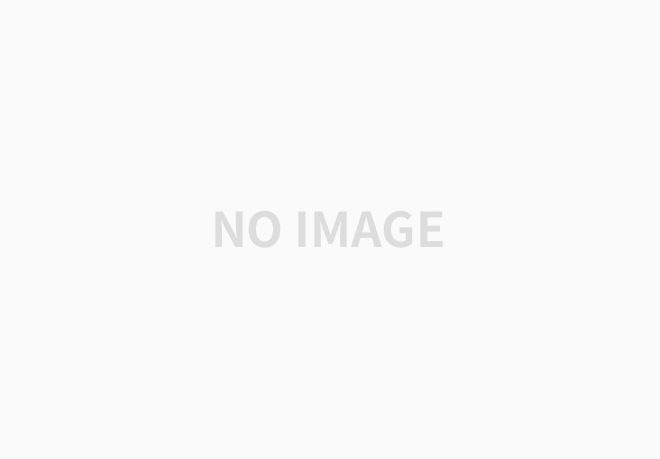
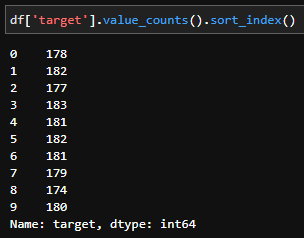
4. Linnerud 체력검정 데이터 (load_linnerud, 다중 회귀 문제)
- 20명의 체력검정 데이터 (Linnerud - 데이터셋 창시자의 이름)
- target 3개: Weight, Waist, Pulse (체중, 허리둘레, 맥박)
- 변수 3개: Chins, Situps, Jumps (턱걸이, 윗몸일으키기, 멀리뛰기)
import pandas as pd
from sklearn.datasets import load_linnerud
linnerud = load_linnerud()
df = pd.DataFrame(data = linnerud.data, columns = linnerud.feature_names)
df[linnerud.target_names] = linnerud.target
print(df.shape)
df.head()
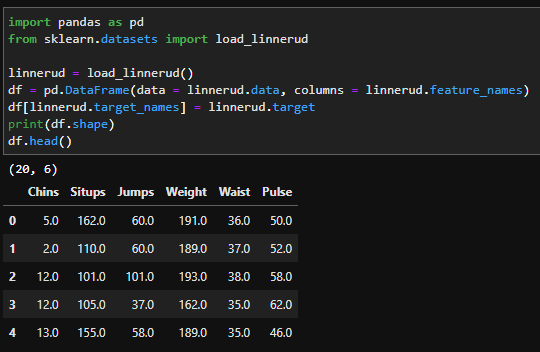
5. 와인 데이터 (load_wine, 분류 문제)
- 와인 등급 분류 데이터
- target 3개: 0, 1, 2
- 변수 13개:
- 'alcohol', 'malic_acid', 'ash', 'alcalinity_of_ash', 'magnesium', 'total_phenols', 'flavanoids', 'nonflavanoid_phenols', 'proanthocyanins', 'color_intensity', 'hue', 'od280/od315_of_diluted_wines', 'proline'
- (알코올 도수, 말산, 잔재, 잔재의 알칼리도, 마그네슘, 총 페놀, 플라보노이드, 비플라보노이드 페놀, 프로안토시아닌, 색상 강도, 색조, 희석 와인의 OD280/OD315, 프롤린)
import pandas as pd
from sklearn.datasets import load_wine
wine = load_wine()
df = pd.DataFrame(wine.data, columns=wine.feature_names)
df['target'] = wine.target
print(df.shape)
df.head()
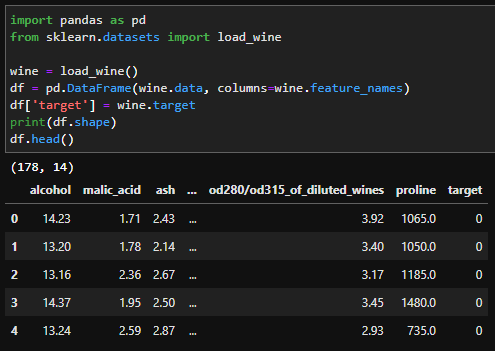
6. 유방암 데이터 (load_breast_cancer, 분류 문제)
- 유방암 여부 분류 데이터
- target 2개: 0 (유방암 X), 1 (유방암 O)
- 변수 30개:
- 'mean radius', 'mean texture', 'mean perimeter', 'mean area', 'mean smoothness', 'mean compactness', 'mean concavity', 'mean concave points', 'mean symmetry', 'mean fractal dimension', 'radius error', 'texture error', 'perimeter error', 'area error', 'smoothness error', 'compactness error', 'concavity error', 'concave points error', 'symmetry error', 'fractal dimension error', 'worst radius', 'worst texture', 'worst perimeter', 'worst area', 'worst smoothness', 'worst compactness', 'worst concavity', 'worst concave points', 'worst symmetry', 'worst fractal dimension'
- (평균 반경, 평균 질감, 평균 둘레, 평균 면적, 평균 매끄러움, 평균 조밀도, 평균 오목함, 평균 오목한 점, 평균 대칭성, 평균 프랙탈 차원, 반경 오차, 질감 오차, 둘레 오차, 면적 오차, 매끄러움 오차, 조밀도 오차, 오목함 오차, 오목한 점 오차, 대칭성 오차, 프랙탈 차원 오차, 최악의 반경, 최악의 질감, 최악의 둘레, 최악의 면적, 최악의 매끄러움, 최악의 조밀도, 최악의 오목함, 최악의 오목한 점, 최악의 대칭성, 최악의 프랙탈 차원)
import pandas as pd
from sklearn.datasets import load_breast_cancer
breast_cancer = load_breast_cancer()
df = pd.DataFrame(breast_cancer.data, columns=breast_cancer.feature_names)
df['target'] = breast_cancer.target
print(df.shape)
df.head()
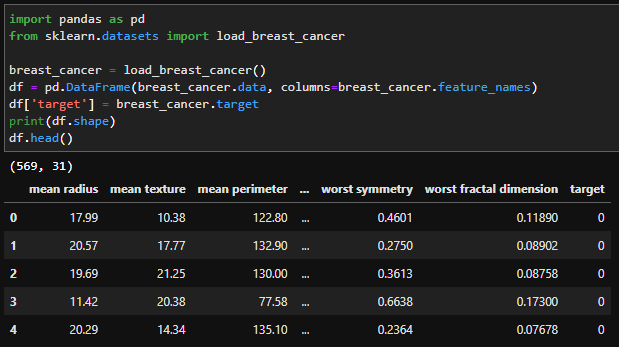

'Python' 카테고리의 다른 글
[Python] 파이썬 Seaborn을 활용한 데이터 시각화 (0) | 2024.05.07 |
---|---|
[작성중] Selenium을 통한 잡플래닛 (Jobplanet) 면접 후기 가져오기 (0) | 2023.03.10 |
[파이썬] 순서 유지하면서 특정 list에 있는 단어만 남기기 (0) | 2021.07.19 |
[파이썬] 문장에서 특정 값 이후부터 삭제 (0) | 2021.07.18 |
[파이썬] 사전 (dictionary)을 통한 단어 바꾸기 (0) | 2021.07.18 |